Appendix B: Methodology
This section provides an overview of the evaluation methodology and data collection approach.
B.1 Overview of the evaluation methodology
The evaluation has been jointly delivered by the DTA and Nous.
A mixed-methods approach was used to evaluate the trial against the 4 trial evaluation objectives. Working with the Australian Centre for Evaluation, DTA designed the evaluation plan and data collection methodology with the pre-use and post-use survey. Nous reviewed and finalised the evaluation plan and assisted with the remaining evaluation activities. Specifically, these included:
- conducting focus groups with trial participants,
- interviewing key government agencies,
- co-designing the post-use survey with the AI in Government Taskforce,
- delivering the post-use survey.
The engagement activities led by Nous complemented engagement conducted by the DTA earlier in the year. Table 8 below details the key activities and milestones of the trial and evaluation.
Start date | End date | Description | Owner |
---|---|---|---|
2023 | |||
7 August | - | AI in Government Taskforce is established | - |
1 November | - | Microsoft 365 Copilot becomes generally available | - |
16 November | - | Trial of Microsoft 365 Copilot is announced | - |
2024 | |||
1 January | 30 June | Microsoft 365 Copilot trial period | - |
January | February | Develop evaluation plan | DTA |
29 February | 11 April | Issue pre-use survey | DTA |
29 February | 19 July | Collate items into issues register | DTA |
7 March | 13 May | Interview DTA trial participants | DTA |
3 May | 17 May | Issue pulse survey | DTA |
13 May | 20 May | Analyse surveys and DTA trial participant interviews | Nous Group |
13 May | 24 May | Develop mid-trial review and interim report | Nous Group |
24 June | 19 July | Conduct focus groups | Nous Group |
2 July | 12 July | Issue post-use survey | DTA |
8 July | 20 July | Analyse qualitative and quantitative data analysed | Nous Group |
8 July | 24 August | Develop final evaluation report | Nous Group |
19 July | 26 July | Review agency reports | Nous Group |
A program logic outlined the intended impacts of the trial.
A theory of change and program logic was developed by the DTA Copilot trial team in consultation with the Australian Centre for Evaluation.
A theory of change describes, at a high level, how program activities will lead to the program’s intended outcomes. The following program logic expands on the theory of change, articulating in more detail the relationship between desired outcomes and the required inputs, activities and outputs.
Inputs
The foundation of the framework consists of inputs, which include:
- staff time and resources
- expenditure
- copilot licences
- Microsoft contract.
Activities
The activities build upon the inputs and are designed to facilitate the use of Copilot:
- Establishing groups in government teams to share experience and learnings with Copilot.
- Establishing Copilot leads in each agency to ensure safe and responsible guidance is shared with users.
- Microsoft providing training sessions on how to use Copilot.
- Microsoft providing access to additional resources (guides and tutorials) and support to address issues.
- Establishing an issues register to collect issues on benefits challenges and barriers to innovation.
Outputs
Outputs are the immediate results of these activities:
- Staff/users are appropriately trained to engage with Copilot.
- Copilot is regularly accessed by staff/users.
- Sentiment is baselined amongst staff/users.
- Ease of use is baselined among staff/users.
- Issues register is actively populated.
Short-term outcomes
These are the direct effects of the outputs in the short term:
- Staff/users have increased confidence in the use of Copilot.
- Improvements to productivity from regular access and use.
- Improved sentiment reported from regular access.
- Unintended outcomes are identified and catalogued.
Medium-term outcomes
The medium-term outcomes reflect the sustained impact of the short-term outcomes:
- Consistent use of Copilot in day-to-day work as an embedded capability.
- Expectations of staff/users are met.
- Treatment actions are scoped for the catalogued unintended outcomes.
Long-term outcomes
The ultimate goals of the framework are the long-term outcomes:
- Improved understanding of Copilot and its safe use.
- More time available for higher priority work.
- Increased staff satisfaction.
- Unintended Copilot outcomes and challenges identified and mitigated.
Key evaluation questions guided data collection and analysis.
Nous developed key evaluation questions to structure the data collection and analysis. The key evaluation questions at Table 9 are based on the 4 evaluation objectives and informed the design of focus groups, the post-use survey and final evaluation report.
Evaluation objective | Key lines of enquiry | |
---|---|---|
Employee related outcomes | Determine whether Microsoft 365 Copilot, as an example of generative AI, benefits APS productivity in terms of efficiency, output quality, process improvements and agency ability to deliver on priorities. | What are the perceived effects of Copilot on APS employees? |
Productivity | Evaluate APS staff sentiment about the use of Copilot. | What are the perceived productivity benefits of Copilot? |
Whole-of-government adoption of generative AI | Determine whether and to what extent Microsoft 365 Copilot as an example of generative AI can be implemented in a safe and responsible way across government. | What are the identified adoption challenges of Copilot, as an example of generative AI, in the APS in the short and long term? |
Unintended outcomes | Identify and understand unintended benefits, consequences, or challenges of implementing Microsoft 365 Copilot, as an example of generative AI, and the implications on adoption of generative AI in the APS. | Are there any perceived unintended outcomes from the adoption of Copilot? Are there broader generative AI effects on the APS? |
B.2 Data collection and analysis approach
A mixed-methods evaluation approach was adopted to assess the perceived impact of Copilot and to understand participant experiences.
A mixed-methods approach – blending quantitative and qualitative data sources – was used to assess the effect of Copilot on the APS during the trial. Quantitative data identified trends on the usage and sentiment of Copilot, while qualitative data provided context and depth to the insights. Three key streams of data collection were conducted:
Document and data review
This involved analysis of existing data such as the Copilot issues register, existing agency feedback, agency-produced documentation e.g. agency Copilot evaluations and research papers.
Consultations
Delivery of focus groups with trial participants and interviews with select government agencies. Insights from DTA interview with participants were also incorporated into the evaluation.
Surveys
Analysis of the pre-use and pulse survey in addition to the design and delivery of a post-use survey.
At least 2,000 individuals, representing more than 50 agencies, contributed to the evaluation through one or more of these methods. Both the participation in the trial and in the evaluation were non-randomised; participants self-nominated to be part of the trial or were identified by their agencies. Efforts were made across the APS to ensure trial participants were representative of the broader APS and a range of experience with generative AI were selected. Further detail on each data stream is provided below.
Document and data review
The evaluation drew on existing documentation prepared by the DTA and participating agencies to supplement findings. DTA and agency research data was used to test and validate insights from the overall evaluation, as well as incorporate the perspective of agencies that had limited participation in the trial. The document and data review can be separated into:
Issues register
Document for participating agencies to submit technical issues and capability limitations of Copilot to the DTA.
Research papers
Documents produced by government entities that investigate the implications of Copilot and generative AI. This included: Office of the Victorian Information Commissioner’s Public statement on the use of Copilot for Microsoft 365 in the Victorian public sector; and the Productivity Commission’s Making the most of the AI opportunity: productivity, regulation and data access.
Internal evaluations
Agency-led evaluations and benefits realisation reports made available to the evaluation team. Agencies that provided their internal results included: Australian Taxation Office (ATO); Commonwealth Scientific and Industrial Research Organisation (CSIRO); Department of Home Affairs (Home Affairs); Department of Industry, Science and Resources (DISR).
Consultations
The evaluation gathered qualitative data on the experience of trial participants through both outreach interviews conducted by the DTA and further focus groups and interviews led by Nous. In total, the evaluation has conducted:
- 17 focus groups across APS job families
- 24 targeted participant interviews conducted by the DTA
- 9 interviews with select government agencies.
Nous’ proprietary generative AI tools were used to process transcripts from interviews and focus groups to identify potential themes and biases in the synthesis of focus group insights. Generative AI supported Nous’s human-led identification of findings against the evaluation’s key lines of enquiry.
Surveys
Three surveys were deployed to trial participants to gather quantitative data about the sentiment and effect of Copilot. A total of 3 surveys were deployed during the trial. There were 1,556 and 1,159 survey responses for the pre-use and pulse survey respectively. In comparison, the post-use survey had 831 responses.
Despite the lower number of total responses in the post-use survey, the sample size was sufficient to ensure 95% confidence intervals (at the overall level) and were within a margin of error of 5%. It is likely agency fatigue with Microsoft 365 Copilot evaluation activities, in conjunction with a shorter survey window, contributed to the lower responses for the post-use survey.
There were 3 questions asked in post-use survey that were originally included in either the pre-use or pulse survey. These questions were repeated to compare responses of trial participants before and after the survey and measure the change in sentiment. These questions were:
Pre-use survey
Which of the following best describes your sentiment about Copilot after having used it?
Pre-use survey
To what extent do you agree or disagree with the following statements: ‘I believe Copilot will…’ / ‘using Copilot has…’
Pulse survey
How little or how much do you agree with the following statement: ‘I feel confident in my skills and abilities to use Copilot.’
The survey responses of trial participants who completed the pre-use and post-use survey and pulse and post-use survey were analysed to assess the change in sentiment over the course of the trial.
Analysis of survey responses have been aggregated to ensure statistical robustness. The following APS job families have been aggregated as shown in Table 10.
Group | Job families |
---|---|
Corporate | Accounting and Finance Administration Communications and Marketing Human Resources Information and Knowledge Management Legal and Parliamentary |
ICT and Digital Solutions | ICT and Digital Solutions |
Policy and Program Management | Policy Portfolio, Program and Project Management Service Delivery |
Technical | Compliance and Regulation Data and Research Engineering and Technical Intelligence Science and Health |
Post-use responses from Trades and Labour, and Monitoring and Audit were excluded from job family-level reporting as their sample size was less than 10. Their responses were still included in aggregate findings. For APS classifications, APS 3-6 have been aggregated.
B.3 Limitations
The representation from APS classifications and job families in engagement activities may not be reflective of the broader APS population.
Given the non-randomised recruitment of trial participants, there is likely an element of selection bias in the results of the evaluation. While the DTA encouraged agencies to distribute Copilot licenses across different APS classifications and job families to mitigate against selection bias, the sample of participants in the trial – and consequently those who contributed to surveys, focus groups and interviews – may not reflect the overall sentiments of the APS.
For APS classifications, there is an overrepresentation of EL1s, EL2s and SES in survey activities. Conversely, there was a lower representation of junior APS classifications (APS 1 to 4) when compared with the proportions of the overall APS workforce. This means that the evaluation results may not be truly capture junior APS views (likely graduates) and disproportionately contain the views of ‘middle managers.’ In addition, the sentiments (in addition to use cases) of APS job families such as Service Delivery may not be adequately captured in the evaluation.
For APS job families, ICT and Digital Solutions and Policy were the 2 most overrepresented in survey responses versus their normal proportion in the APS. Service Delivery was the only job family that had significant underrepresentation in the surveys, comprising only around 5% of the survey responses but represent around 25% of the APS workforce.
Appendix D provides a detailed breakdown of pre-use and post-use survey participation by APS classifications and job families compared to the entire APS population.
There is likely a positive bias sentiment amongst trial participants.
It is likely that trial participants and as a subset, participants in surveys and focus groups, have a positive biased sentiment towards Copilot compared to the broader APS. As shown in Figure 16, the majority of pre-use survey respondents were optimistic about Copilot heading into the trial (73%) and were familiar with generative AI before the Trial (66%).
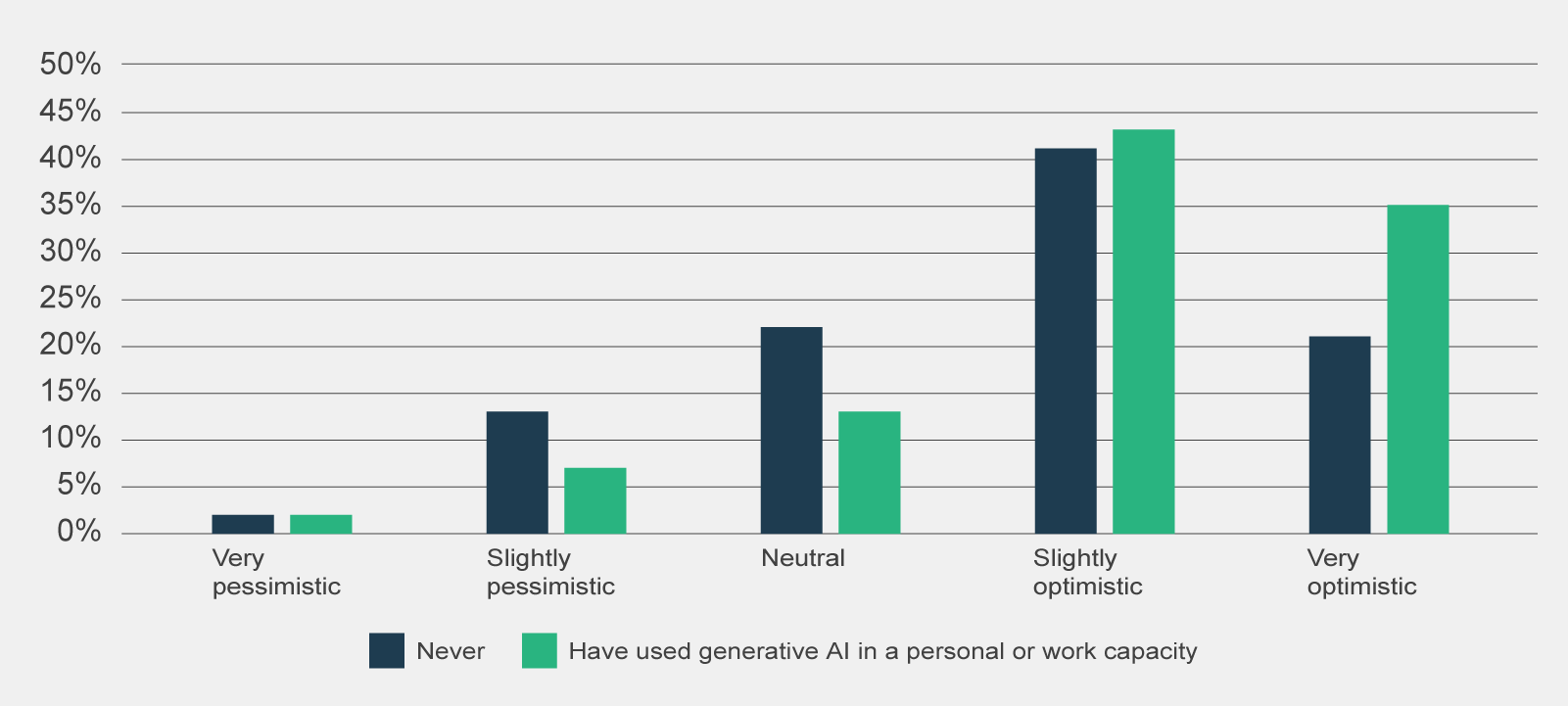
There was an inconsistent rollout of Copilot across agencies.
The experience and sentiment of trial participants may be affected by when their agency began participating in the trial and what version of Copilot their agency provided.
On the former, agencies received their Copilot licences between 1 January to 1 April 2024. Some agencies opted to distribute Copilot licences to participants later in this period once internal security assessments were complete. This meant that agencies participating in the trial had different timeframes to build capability and identify Copilot use cases, which could potentially affect participants’ overall sentiment and experience with Copilot.
Further, agencies who joined the trial later may not have been able to contribute to early evaluation activities, such as the pre-use survey or initial interviews, therefore excluding their perspective and preventing later comparison of outcomes.
On the latter, since the trial began, Microsoft has released 60 updates for Copilot to enable new features – including rectifying early technical glitches. Due to either information security requirements or a misalignment between agency update schedules, the new features of Copilot may have been inconsistently adopted across participating agencies or at times, not at all.
This means that there could be significant variability with Copilot functionality across trial participants and it is difficult for the evaluation to discern the extent to which participant sentiments are due to specific agency settings or Copilot itself.
Trial participants expressed a level of evaluation fatigue.
Agencies were encouraged to undertake their own evaluations to ensure the future adoption of Copilot or generative AI reflected their agency’s needs. Many participating agencies conducted internal evaluations of Copilot that involved surveys, interviews and productivity studies.
Decreasing rates of evaluation activity participation over the trial indicates that trial participants may have become fatigued from evaluation activities. The survey response rate progressively decreased across the pre-use to pulse to post-use surveys. Lower response rates in the post-use survey (n = 831) and for those who completed both the pre-use and post-use survey (n = 330) may impact how representative the data is of the trial population. Participation in the Nous-facilitated focus groups and the post-use survey was impacted by these parallel initiatives and the subsequent evaluation fatigue.
This means that the evaluation may not have been able to engage with a wide range of trial participants with a proportion of trial participants opting to only provide responses to their own agency evaluation. This may have been mitigated to a degree with some agencies sharing their results to the evaluation.
The impact of Copilot relied on trial participants’ self-assessment of productivity benefits.
The evaluation methodology relies on the trial participants’ self-assessed impacts of Copilot which may naturally under or overestimate impacts – particularly time savings. Where possible, the evaluation has compared its productivity findings against other APS agency evaluations and external research to verify the productivity savings put forth by trial participants.
Nevertheless, there is a risk that the impact of Copilot – in particular the productivity estimates from Copilot use, may not accurately reflect Copilot’s actual productivity impacts.